Generative AI in Biology Market Forecasted to Boost USD 346.9 Mn | CAGR of 17.5% by 2032
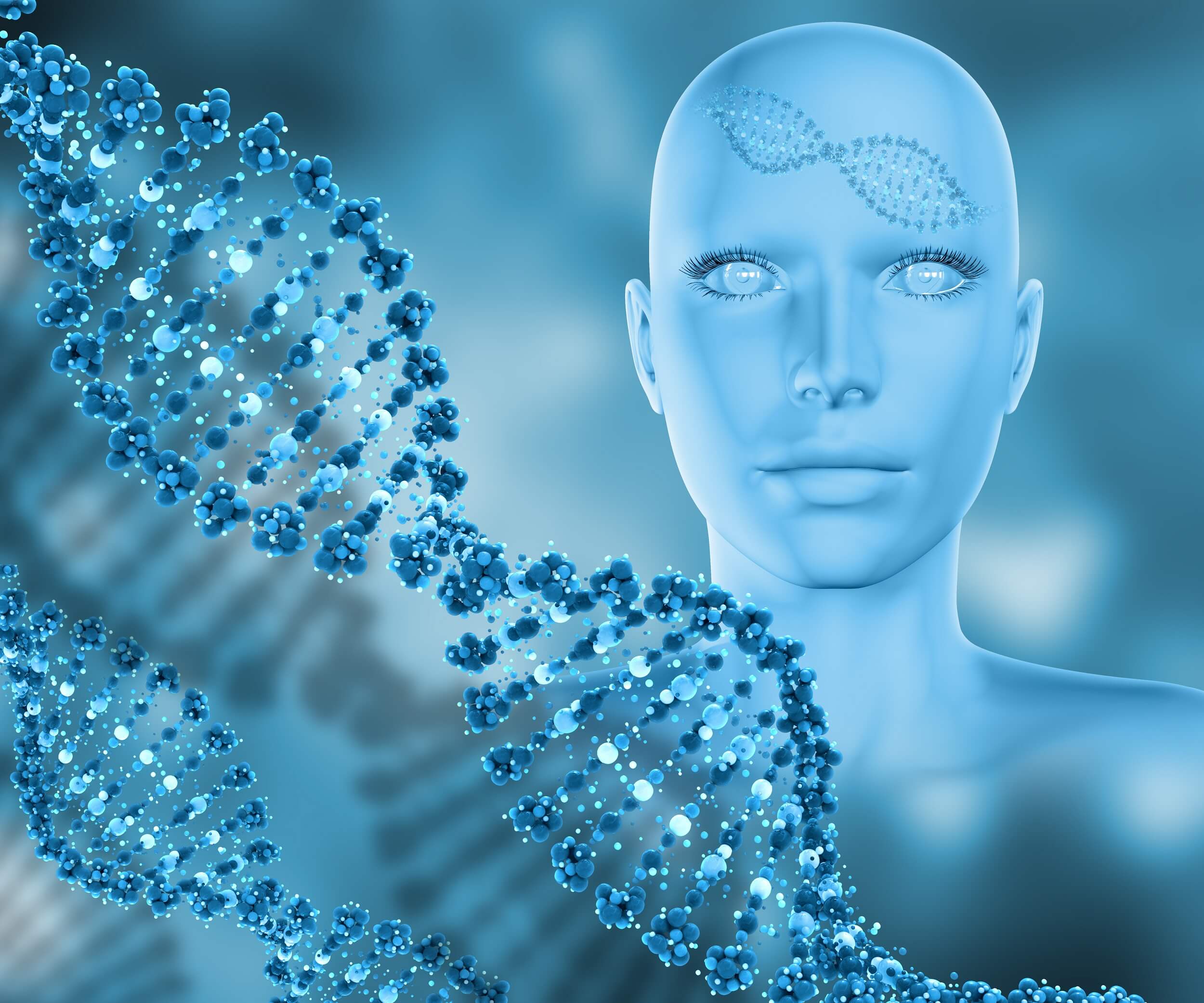
Page Contents
Market Overview
Published Via 11Press : Generative AI in Biology Market size is expected to be worth around USD 346.9 Mn by 2032 from USD 72.0 Mn in 2022, growing at a CAGR of 17.5% during the forecast period from 2022 to 2032.
Biomedicine has witnessed tremendous advances over the past several years, and one area that has demonstrated, particularly great promise is generative artificial intelligence (AI). Generative AI refers to using machine learning algorithms to generate unique and new data derived from existing datasets in ways similar to what was learned previously – making this technology invaluable across various biological domains, from drug discovery and protein engineering through genomics and personalized medicine.
Generative AI has revolutionized drug discovery. By training on large databases of molecular structures and their associated properties, AI algorithms are capable of quickly creating molecules with desirable characteristics – speeding up drug discovery while potentially cutting costs and time associated with traditional approaches.
Generative AI holds tremendous promise to revolutionize our understanding of genomics. By processing vast amounts of genomic data, AI models can detect patterns and relationships that humans would miss; this may lead to new genetic markers for disease detection as well as insights into complex regulatory networks as well as better insight into genetic variations between populations.
Generative AI offers great potential in personalized medicine. By analyzing patient-specific data such as genomic sequence, electronic health records and lifestyle factors, AI algorithms can generate tailored treatment recommendations tailored specifically to individual patients – helping healthcare providers deliver more precise and effective care while also decreasing healthcare costs.
Generative AI has also found application in protein engineering, where it allows for the design of proteins with new functions and properties. By training on vast protein databases and employing deep learning algorithms, AI models can generate new protein sequences which can then be synthesized and tested in laboratories; this has the potential to revolutionize fields such as enzyme design, biofuel production, and biopharmaceutical development.
The market for generative AI in biology is experiencing unprecedented growth, with both startups and established companies investing heavily in this technology. Pharmaceutical firms are working with AI firms to speed up drug discovery processes while genomics companies use generative AI to analyze human genome sequence data. Furthermore, academic research institutions are actively exploring its use across a wide variety of biological domains.
However, challenges still exist: ethical considerations surrounding AI use in biology must be addressed, including privacy concerns and data bias; further investigation must also take place regarding the interpretability and reliability of generative AI models in a biological context.
Request Sample Copy of Generative AI in Biology Market Report at: https://marketresearch.biz/report/generative-ai-in-biology-market/request-sample
Key Takeaways
- Generative AI offers a revolutionary method of drug discovery, drastically cutting time and costs associated with it.
- Generative AI applied to genomics can uncover hidden patterns and genetic markers for diseases, providing us with greater insights into our human genome.
- Generative AI brings personalized medicine a step closer by offering tailored treatment recommendations based on patient data.
- Protein engineering can benefit greatly from artificial intelligence (AI), enabling designers to design proteins with specific functions and properties.
- Generative AI for biology research is experiencing exponential growth, as both startups and established firms alike invest in this cutting-edge technology.
- Partnerships between pharmaceutical companies and AI firms are increasing adoption of generative AI for drug discovery.
- Ethical considerations must be addressed for the responsible use of generative AI in biology. Privacy and data bias need to be considered.
- Interpretability and reliability of generative AI models used to model biological phenomena require further examination.
- Academia plays an integral part in exploring the applications of Generative AI across diverse biological domains, leading to innovation in this space.
Regional Snapshot
- North America and particularly the United States is a center for generative AI applications in biology, with numerous companies, research institutions, and academic centers all dedicated to creating and applying this technology for drug discovery, genomics analysis, and personalized medicine applications.
- European nations such as the UK, Germany and France are showing increasing interest in artificial intelligence applications to biology. Academic institutions and research centers collaborate with industry players on drug discovery efforts such as genomics research or precision medicine initiatives. European regulations place special importance on ethical considerations and data privacy regulations.
- Asia-Pacific countries such as China, Japan, and South Korea are witnessing notable advancements in generative AI biology research. Academic and industrial collaborations drive research in areas like drug discovery, genomics, and biotechnology; with its large population providing rich resources for genomic data analysis and personalized medicine applications.
- Latin America is emerging as an exciting region for generative AI applications in biology. Countries like Brazil, Mexico and Argentina have established research centers and partnerships dedicated to exploring AI applications in drug discovery, genomics and precision medicine – with an increasing emphasis on using this technology to address regional health challenges.
- Middle East and Africa are slowly adopting artificial intelligence (AI) technologies in biology research, with notable contributions made by Israel and South Africa in genomics research and drug discovery. Furthermore, attempts are being made to leverage AI for personalized medicine solutions that enhance healthcare outcomes in these regions.
For any inquiries, Speak to our expert at: https://marketresearch.biz/report/generative-ai-in-biology-market/#inquiry
Drivers
Advances in Computational Power
Computing power advancement and availability of high-performance computing resources has contributed significantly to the expansion of generative AI in biology. Being able to rapidly process large datasets and complex algorithms at a faster pace allows more sophisticated and accurate AI models that improve the performance of applications utilizing this type of AI in different biological fields.
Big Data and Access to Datasets
The proliferation of biological data such as genomic sequences, protein structures and clinical records provides a rich source for training generative AI models. Such vast and diverse datasets enable AI algorithms to learn patterns quickly and produce novel outputs in drug discovery, genomics and personalized medicine – driving its use across biology.
Accelerating Drug Discovery
Traditional drug discovery processes are time-consuming, expensive and rarely result in successful drugs. Generative AI in biology offers a promising solution by quickly creating new molecules with desired properties – this accelerates drug discovery processes while simultaneously cutting costs while uncovering promising candidates that would have otherwise gone undetected using traditional approaches.
Personalized Medicine and Precision Healthcare
Personalized medicine – in which treatments are tailored specifically for each individual – is rapidly expanding. Generative AI plays an essential role in this trend by analyzing genomic, medical record and lifestyle factors of patients to generate personalized recommendations. This method may improve patient outcomes and revolutionize healthcare delivery systems.
Restraints
Ethical Considerations
Generative AI integration into biology raises ethical concerns related to privacy, data security and potential misuse. Therefore it is vitally important that robust ethical guidelines and regulations be put in place in order to ensure responsible usage, safeguard sensitive data as well as manage any unintended or undesirable results of using AI-generated solutions.
Interpretability and Transparency
Generative AI models can be complex, making it challenging to interpret their decision-making processes and comprehend their underlying logic. Without interpretability or transparency, trust and acceptance in critical applications such as drug discovery and healthcare may suffer due to inexplicable decisions without sufficient explanations and justifications for decisions taken by such AI programs.
Data Quality and Bias
The quality, representativeness and bias present in training data used for generative AI models can have an enormous impact on their outputs. Biases in data, such as underrepresentation of certain populations or skewed datasets can lead to biased or inaccurate results; to successfully implement generative AI in biology it is therefore paramount that high-quality, diverse training data be produced that allows generative AI applications.
Regulative and Legal Frameworks of Operations
Regulatory landscape surrounding generative AI technologies for biology research remains ever-evolving, creating challenges in terms of compliance and legal requirements. Therefore, creating suitable frameworks and guidelines regarding intellectual property rights, data ownership, privacy regulations and safety considerations are necessary in order to facilitate responsible and ethical usage.
Opportunities
Enhance Drug Discovery and Development
Generative AI offers unparalleled possibilities for speedier and more cost-effective drug discovery and development processes. By synthesizing new molecules with enhanced properties, AI algorithms can identify potential candidates with greater success rates while cutting time and costs associated with traditional approaches.
Precision Medicine and Customized Therapies
Integrating AI into biology enables personalized medicine approaches by harnessing patient data. This opens up opportunities for developing targeted therapies, optimizing treatment plans, and improving healthcare outcomes by tailoring treatments according to an individual patient's specific characteristics, leading to more efficient and effective healthcare delivery.
Biotechnology and Protein Engineering
Generative AI can transform biotechnology by designing proteins with specific functions and properties. This opens up opportunities to design enzymes, biofuels and therapeutic proteins with unique functions and properties. AI models can generate protein sequences which can then be synthesized and tested, speeding up discovery of innovative biotechnological solutions.
Collaborative Research and Industry Partnerships
As interest in generative AI in biology has expanded, so has its application across academia, industry and research institutions. Partnerships facilitate knowledge transfer between disciplines as well as access to diverse datasets and joint research initiatives in this space – driving innovation forward in biology research. Collaborative initiatives offer opportunities for sharing expertise, validating AI models and translating research findings into practical applications.
Take a look at the PDF sample of this report: https://marketresearch.biz/report/generative-ai-in-biology-market/request-sample
Challenges
Data Integrity and Integration Solutions Provided
Even with all of this biological data readily available, accessing and integrating different datasets from disparate sources remains a challenging endeavor. Data silos, varied formats, and privacy concerns prevent seamless data utilization for training generative AI models; developing standard sharing mechanisms while taking measures to address privacy concerns is therefore key for realizing the full potential of generative AI in biology.
Model Generalization and Robustness
Generative AI models must generalize well to novel and untried data in order to be effective. Ensuring robustness and reliability can be challenging when handling variations, noise and outliers; developing robust algorithms capable of adapting to various biological contexts and datasets is key for widespread adoption of generative AI in biology.
Explainability and Trust
Generative AI models often struggle to gain trust and acceptance among end-users, regulatory bodies, and the general public. Therefore, creating AI models with sufficient transparency for end users, regulators, and the general public alike is crucial in order to foster ethical deployment within sensitive fields like healthcare.
Resource Constraints
Implementing Generative AI requires significant computational resources, including high-performance computing infrastructure and storage capacity. Affordability may present issues for smaller research institutions and startups. Therefore, developing efficient algorithms which operate under resource constraints is key for wider adoption and accessibility of Generative AI in biology.
Market Segmentation
Based On Application
- Medical Imaging
- Genomics and Proteomics
- Drug Discovery and Development
- Protein Engineering
- Synthetic Biology
- Other Applications
Based On Technology
- Generative Adversarial Networks
- Variational Autoencoders
- Reinforcement Learning
- Other Technologies
Based On End-User
- Pharmaceutical and Biotechnology Companies
- Research Institutions
- Healthcare Provider
- Other End-Users
Key Players
- NVIDIA Corporation
- IBM Corporation
- BenevolentAI
- DeepMind Technologies Limited
- Insilico Medicine
- Recursion Pharmaceuticals
- Zymergen
- Other Key Players
Report Scope
Report Attribute | Details |
Market size value in 2022 | USD 72.0 Mn |
Revenue Forecast by 2032 | USD 346.9 Mn |
Growth Rate | CAGR Of 17.5% |
Regions Covered | North America, Europe, Asia Pacific, Latin America, and Middle East & Africa, and Rest of the World |
Historical Years | 2017-2022 |
Base Year | 2022 |
Estimated Year | 2023 |
Short-Term Projection Year | 2028 |
Long-Term Projected Year | 2032 |
Request Customization Of The Report: https://marketresearch.biz/report/generative-ai-in-biology-market/#request-for-customization
Recent Developments
- In 2020, DeepMind's AlphaFold algorithm made waves in 2020 for successfully predicting protein structures – an extremely difficult challenge in biology. This remarkable feat had wide-reaching ramifications for drug discovery and protein engineering alike as it provided vital insight into both structure and function of proteins.
- In 2021,Researchers at MIT used artificial intelligence models to generate novel antibiotics. The AI system analyzed large databases of chemicals that had antimicrobial activities against bacteria, leading to the identification of promising new candidates as potential antibacterials.
- In 2023, Berg Health, a biopharmaceutical company, announced their collaboration with AI firm GNS Healthcare to combine generative AI and machine learning with clinical data for drug discovery acceleration and precision therapy development.
- In March 2023, Insilico Medicine raised $250 million through their Series C funding round. Their cutting-edge artificial intelligence algorithms use machine learning technology to identify novel drug targets, optimize molecules, predict potential drug toxicity risks and accelerate drug discovery processes, making their discovery process faster than ever.
FAQ
1. What is Generative AI in Biology?
A. Generative AI in biology refers to using artificial intelligence algorithms to generate unique, unrelated data for biological fields such as drug discovery, genomics and personalized medicine.
2. How is Generative AI beneficial to drug discovery?
A. Generative AI accelerates drug discovery by creating molecules with desired properties quickly, helping identify potential drug candidates more quickly, and decreasing time and cost associated with traditional methods of discovery.
3. What are the advantages of using AI for genomics research?
A. Generative AI in genomics provides an effective means of analyzing vast amounts of genomic data, leading to the identification of genetic markers for diseases, insights into regulatory networks and advancements in personalized medicine.
4. How does Generative AI facilitate personalized medicine?
A. Generative AI uses patient data to make tailored treatment recommendations, improving both precision and efficacy in personalized medicine approaches.
5. What are the challenges associated with implementing generative AI in biology?
A. Implementing generative AI in biology presents many unique challenges, including ethical considerations, interpretability of AI models, data quality concerns and regulatory frameworks governing privacy and bias issues.
6. How is Generative AI driving innovation in biotechnology?
A. Generative AI allows for the design of novel proteins with specific functions, advancing fields such as enzyme design, biofuel production and therapeutic protein development in biotechnology.
7. What can we expect in terms of future developments for generative AI in biology?
A. Future prospects of generative AI in biology look promising, as increased computational power leads to better performance and faster processes, improved healthcare outcomes and groundbreaking discoveries across various life science fields. Ethical and regulatory considerations will remain at the core of its development.
Contact us
Contact Person:ย Mr. Lawrence John
Marketresearch.Biz
Tel:ย +1 (347) 796-4335
Send Email:ย [email protected]
Content has been published via 11press. for more details please contact at [email protected]

The team behind market.us, marketresearch.biz, market.biz and more. Our purpose is to keep our customers ahead of the game with regard to the markets. They may fluctuate up or down, but we will help you to stay ahead of the curve in these market fluctuations. Our consistent growth and ability to deliver in-depth analyses and market insight has engaged genuine market players. They have faith in us to offer the data and information they require to make balanced and decisive marketing decisions.