Generative AI in Biotech Market Is Projected To Grow At A 24.9%
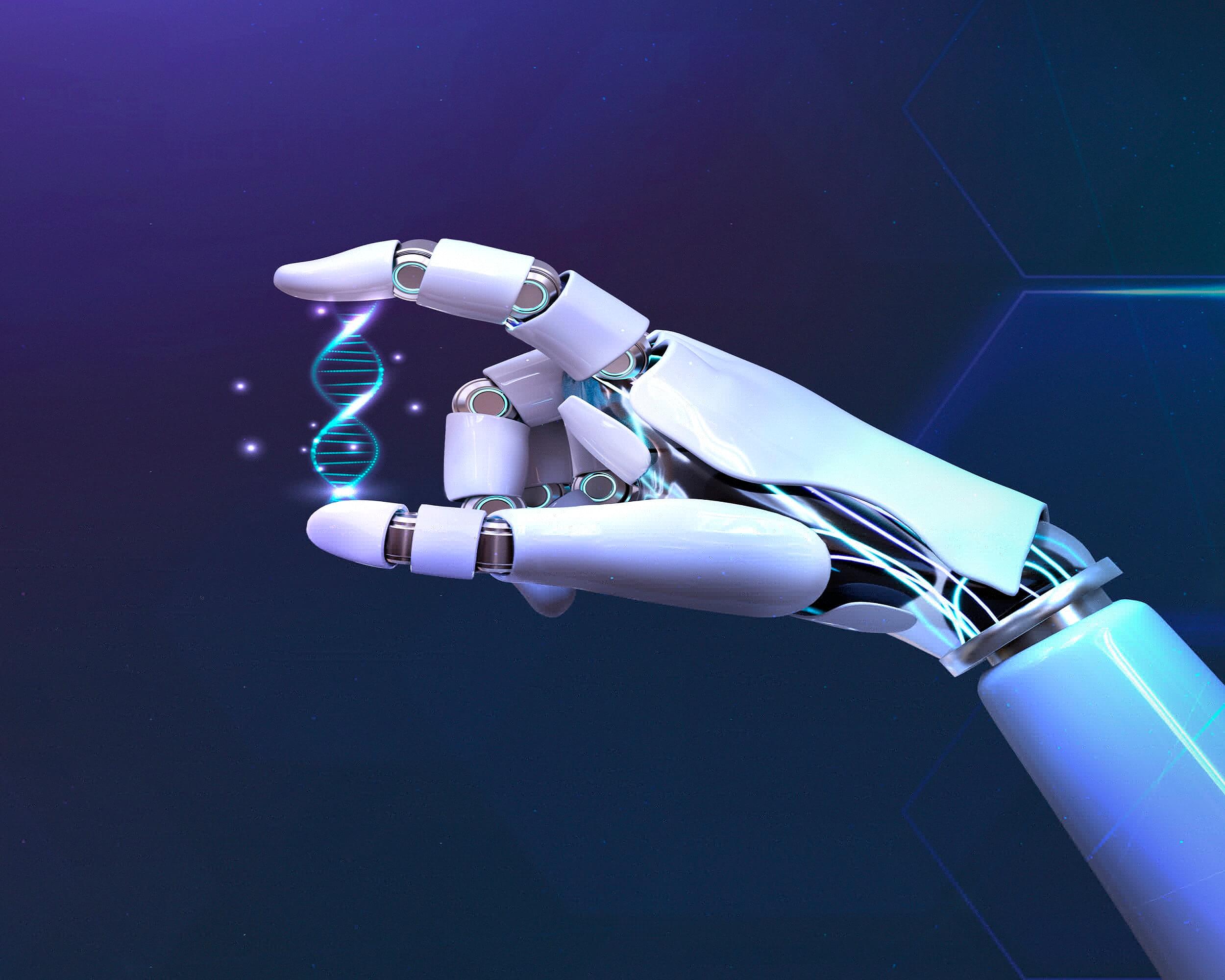
Page Contents
Market Overview
Published Via 11Press : Generative AI in Biotech Market size is expected to be worth around USD 472 Mn by 2032 from USD 54 Mn in 2022, growing at a CAGR of 24.9% during the forecast period from 2022 to 2032.
Generational artificial intelligence (AI) in biotech has received significant attention. Generative AI's ability to simulate and generate novel molecules, proteins and genetic sequences offers great promise for drug discovery, protein engineering and personalized medicine applications. Generative AI may prove revolutionary for drug design processes resulting in faster and more cost-efficient processes than ever before.
Generative AI algorithms use deep learning techniques and large datasets to produce new molecules and genetic sequences with desired properties, using deep learning. By employing this form of Artificial Intelligence (AI), researchers can speed up drug discovery while decreasing costs and time-to-market for new therapies.
Generative AI has made significant strides forward in drug discovery. Pharmaceutical companies are using this type of AI to quickly generate virtual libraries of compounds with potential interactions for specific disease targets, which researchers then screen millions of virtual compounds quickly before selecting promising candidates for further validation experiments and speeding up the discovery pipeline.
Protein engineering is another field where generative AI can play an essential role. By designing proteins with specific functions or properties, researchers can create novel enzymes, antibodies, and other biologics for therapeutic use. Generative AI algorithms facilitate exploration of vast sequences and structural spaces, leading to discovery of proteins with increased stability, specificity or activity which have the potential to revolutionize fields such as enzyme catalysis, biocatalysis and antibody engineering.
Generative AI plays an integral part in personalized medicine. By analyzing patient data and genetic information, AI algorithms can produce tailored treatment plans tailored specifically to an individual's unique characteristics – this enables healthcare providers to optimize treatment strategies, predict drug responses and minimize adverse reactions leading to more efficient and tailored healthcare for every individual patient.
Although Generative AI holds immense potential in biotech, its implementation presents some unique challenges. Data availability and quality are of utmost importance as Generative AI algorithms rely on large, diverse datasets for training purposes. Privacy issues as well as data-sharing agreements must also be carefully considered in order to guarantee ethical use of patient information.
Interpretability and explainability are also active areas of investigation for AI models. Understanding how AI algorithms generate specific sequences or molecules is paramount for their acceptance in regulatory or clinical environments.
Request Sample Copy of Generative AI in Biotech Market Report at: https://marketresearch.biz/report/generative-ai-in-biotech-market/request-sample
Key Takeaways
- Generative AI is revolutionizing the biotech industry by speeding drug discovery and cutting costs.
- Virtual compound libraries created using AI algorithms help in the identification of potential drug candidates.
- Protein engineering can benefit greatly from using generative AI by designing proteins with enhanced functions and properties.
- Personalised medicine is being enhanced through AI-generated treatment plans generated using patient data.
- Data availability and quality are of utmost importance in order to train generative AI models effectively.
- Privacy concerns and ethical considerations must be taken into account in regards to using patient data.
- Interpretability and explainability are ongoing challenges of AI models.
- Generative AI could provide healthcare organizations with an innovative tool for optimizing healthcare strategies and anticipating drug responses.
- Collaboration between AI experts and biotech researchers is integral for the widespread implementation of generative AI in biotechnology markets.
Regional Snapshot
North America and, specifically the US, has long been at the forefront of biotech's adoption and development of generative AI. Top research institutions, well-established biotech firms, and an accommodating regulatory environment all contribute to significant advancements in this area. Companies throughout North America use AI for drug discovery, protein engineering, and personalized medicine applications – several successful ones already making their way into clinical trials!
Europe has emerged as an important region for generative AI applications in biotech. Countries like the UK, Germany and Switzerland boast strong biotech ecosystems and invest heavily in AI research and development. Collaborations between academic institutions, biotech companies and AI startups have fostered innovation in drug discovery, precision medicine and bioinformatics applications in this region. Regulatory bodies across Europe have also taken proactive steps in providing guidelines regarding AI-driven technologies used within healthcare.
Asia Pacific is witnessing rapid expansion of generative AI's use in biotech. Countries like China, Japan and South Korea have made significant investments in AI research and development which has created a booming biotech sector in these regions. Generative AI is being utilized in drug discovery, biomarker identification and personalized medicine initiatives; further bolstered by Asia's large population which provides ample genetic and clinical data analysis potential enhancing this field's growth within this region.
Latin America is gradually adopting generative AI solutions in biotech. Countries like Brazil and Mexico have made great strides toward creating AI-powered solutions for drug discovery and precision medicine. Latin America boasts rich biodiversity that serves as a fertile ground for natural product research; however, limited infrastructure, funding and collaborations pose serious barriers to wider implementation of generative AI technologies.
Middle East and African biotech is only just beginning to embrace generative AI applications; countries such as Israel and South Africa have taken the lead in leading this innovation with Israel being particularly innovative when it comes to AI research and development efforts; AI applications used for genomics research, drug discovery, personalized medicine are gaining ground with partnerships among academic institutions, healthcare organizations and industry players supporting these endeavors. More investment needs to be made into infrastructure and research capabilities in order to accelerate its growth further in these regions.
For any inquiries, Speak to our expert at: https://marketresearch.biz/report/generative-ai-in-biotech-market/#inquiry
Drivers
Technological Advancements
Rapid advances in generative AI technologies such as deep learning algorithms and computational power are driving biotech's use of generative AI. These advancements make generative AI applications more efficient in producing molecules, proteins and genetic sequences quickly and accurately – speeding up drug discovery processes as well as protein engineering processes.
Accelerated Drug Discovery Services
Generative AI helps accelerate drug discovery by rapidly screening millions of potential candidates. This enables researchers to more efficiently identify promising drug candidates, leading to decreased costs and shorter development timelines. Furthermore, its ability to explore vast chemical spaces while anticipating target interactions improves efficiency and success rates of drug discovery efforts.
Potential of Customized Medicine Treatment
Generative AI holds the promise to revolutionize personalized medicine by analyzing patient data and genetic information. AI algorithms can generate personalized treatment plans tailored specifically for each individual that optimize therapies, predict drug responses and minimize adverse reactions – leading to more targeted healthcare interventions that lead to improved patient outcomes and tailored treatment strategies.
Collaboration and Industry Support
As AI experts, biotech researchers, and pharmaceutical companies collaborate more, the rise of generative AI adoption is being fuelled by collaboration among all these stakeholders. Their combined resources enable robust AI models, access to diverse datasets, validation of generated molecules or proteins generated, plus government funding of research in this field – driving its adoption across biotech.
Restraints
Data Accessibility and Quality Assessment Services
Generative AI relies on large and diverse datasets for training purposes. Unfortunately, accessing high-quality, well-annotated biotech data is often challenging to come by, which impedes the development and performance of generative AI models, impacting their accuracy and reliability.
Regulatory Challenges
The regulatory landscape surrounding biotech's use of generative AI is still emerging, making compliance with existing regulations such as data privacy and ethical considerations challenging. Regulators face issues related to data sharing, patient privacy and interpretability of AI models; meeting these concerns and setting clear guidelines and standards will facilitate responsible and widespread adoption.
Interpretability and Explainability
Generative AI models present a significant challenge when it comes to interpretability and explainability, making acceptance in regulatory or clinical environments essential. Explaining rationale behind generated outputs as well as decision-making processes is integral in creating trust with biotech users and ultimately accepting generative AI in biotech applications.
Computational Resources and Scalability
Generative AI algorithms require substantial computational resources for training and inference. Modeling chemical and biological spaces is computationally intensive and time-consuming; scaling these algorithms up to handle larger datasets or complex problems remains challenging. High-performance computing infrastructure along with efficient algorithms capable of handling this scale and complexity is vital in order to overcome this roadblock.
Opportunities
Target Identification Capabilities Improve
Generative AI provides researchers with an excellent tool to explore novel target spaces and discover potential drug targets. By synthesizing molecules that interact with specific disease targets, researchers can discover new therapeutic possibilities and broaden the scope of drug discovery – opening doors to meeting unmet medical needs and testing out innovative treatment methodologies.
Collaborations with AI Startups
Biotech companies have recently seen increased collaborations with Artificial Intelligence (AI) startups specializing in generative AI. These startups bring expertise and innovation in AI technologies and algorithms that allow biotech firms to tap their capabilities for drug discovery, protein engineering and personalized medicine applications. Collaborating with AI startups can speed research and development efforts while creating disruptive breakthroughs.
Integrating Multiple Omics Data Sources
Generative AI can combine diverse omics data sets, such as genomics, proteomics and metabolomics to generate comprehensive insights. By combining and analyzing information at various biological levels simultaneously, researchers can gain a better understanding of complex biological systems as well as identify potential therapeutic targets or biomarkers. Integrating multi-omics data through generative AI could open new discoveries that lead to precision medicine.
Repurposing Existing Drugs for Alternative Uses
Generative AI can be used to repurpose existing drugs for new indications. By producing modified versions or combinations of known medications, researchers can explore alternative uses and potentially find effective treatments for various diseases. This approach reduces time and cost associated with traditional drug development as safety profiles of repurposed medications are already known.
Take a look at the PDF sample of this report: https://marketresearch.biz/report/generative-ai-in-biotech-market/request-sample
Challenges
Ethical Considerations
Generative AI's ethical considerations in biotech are immense and require careful thought when being applied in practice, particularly data privacy, consent, and fair representation issues. Ensuring responsible use of patient data while eliminating biases from algorithmic decision-making processes are significant obstacles that must be surmounted for widespread implementation of Generative AI solutions in biotech.
Validation and Experimental Verification
Verifying the results of generative AI models experimentally remains an immense challenge. While AI algorithms can generate potential molecules or proteins, proper experimental verification is required to assess their safety, efficacy, and feasibility – thus closing the gap between AI-generated outputs and real world experimental validation for seamless biotech research and development processes.
Limited Generalizability
Generative AI models present a challenge in the biotech industry, as models trained on particular datasets may struggle to adapt to novel or rare data instances. To meet this hurdle, more robust and transferable models that can handle various biological and chemical contexts need to be created so as to extend beyond training data for application beyond their training set.
Regulatory Approval and Acceptance
Generative AI technologies must gain regulatory approval and market acceptance from governing bodies and industry stakeholders. Demonstrating safety, reliability, and efficacy is paramount for gaining regulatory clearance and market acceptance of AI-generated molecules or therapies; collaboration among regulatory agencies, researchers, and industry experts in setting guidelines and standards to validate and approve such biotech solutions is of vital importance in this respect.
Market Segmentation
Based on Technology
- Generative Adversarial Networks (GANs)
- Variational Auto Encoders (VAEs)
- Reinforcement Learning
- Natural Language Processing (NLP)
- Other
Based on Application
- Drug discovery
- Protein engineering
- Genomics
- Bioinformatics
- Other
Based on Organization Type
- Pharmaceutical companies
- Biotechnology startups
- Academic institutions
- Research organizations
- Other
Key Players
- Insilico Medicine
- Recursion Pharmaceuticals
- Atomwise
- Deep Genomics
- BenevolentAI
- Numerate
- Ginkgo Bioworks
- Zymergen
- OpenAI
- DeepMind
- Other
Report Scope
Report Attribute | Details |
Market size value in 2022 | USD 54 Mn |
Revenue Forecast by 2032 | USD 472 Mn |
Growth Rate | CAGR Of 24.9% |
Regions Covered | North America, Europe, Asia Pacific, Latin America, and Middle East & Africa, and Rest of the World |
Historical Years | 2017-2022 |
Base Year | 2022 |
Estimated Year | 2023 |
Short-Term Projection Year | 2028 |
Long-Term Projected Year | 2032 |
Request Customization Of The Report: https://marketresearch.biz/report/generative-ai-in-biotech-market/#request-for-customization
Recent Developments
- In 2023, XtalPi announced an expansion of their generative AI capabilities to include de novo drug design and lead optimization using deep learning algorithms and quantum mechanics, providing novel candidates quickly while accurately predicting their properties – speeding up drug development processes while shortening timelines.
- In 2021, Insilico Medicine made considerable advances with its AI-powered drug discovery efforts by employing generative AI. They announced their partnership with Boehringer Ingelheim to identify targets through their AI platform.
- In 2022, Deep Genomics recently received a significant funding round to expand its artificial intelligence (AI)-powered platform for identifying genetic variants and creating personalized therapies. Their system utilizes deep learning algorithms integrated with genomic data in order to predict mutation impacts and provide potential treatment plans.
FAQ
1. What is Generative AI in Biotech?
A. Generative AI in biotech refers to the application of artificial intelligence algorithms, specifically generative models, to simulate, generate, or optimize molecules, proteins, or genetic sequences. This technology allows researchers to explore vast chemical and biological spaces quickly; ultimately speeding drug discovery, protein engineering, and personalized medicine.
2. How does Generative AI contribute to drug discovery?
A. Generative AI algorithms create virtual compound libraries and screen millions of potential drug candidates, speeding up identification of promising drug candidates while cutting costs and shortening development timelines. Furthermore, this technology increases efficiency in target identification, lead optimization, and the prediction of drug-target interactions.
3. Can Generative AI Be Used for Customized Medicine?
A. Yes, generative AI plays an essential role in personalized medicine. By analyzing patient data such as genomics and clinical information, AI algorithms can generate personalized treatment plans tailored specifically to an individual patient, helping optimize therapies, predict drug responses more precisely and reduce adverse reactions resulting in more tailored and effective healthcare interventions.
4. What are the challenges associated with using generative AI in biotech?
A. Challenges faced in AI include data availability and quality, regulatory considerations, AI model interpretability/explainability/computing resources constraints. Accessing diverse and well-annotated datasets; addressing privacy concerns; setting regulatory guidelines; as well as improving interpretability are ongoing obstacles within this field.
5. How is collaboration fuelling the adoption of generative AI in biotech?
A. AI experts, biotech researchers, and pharmaceutical companies are joining forces to facilitate the widespread adoption of generative AI. Through collaboration they can create robust AI models, gain access to diverse datasets, validate generated molecules or proteins and accelerate R&D efforts. Partnerships with generative AI startups may further speed this up.
6. Are we using generative AI beyond drug discovery?
A. Yes, generative AI is being applied beyond drug discovery. For example, protein engineering uses it to design novel enzymes, antibodies and biologics with improved properties. Generative AI also contributes to personalized medicine applications like biomarker discovery or repurposing existing drugs for new indications – among others.
7. How is Generative AI Regulated in Biotech Industry?
A. Regulatory frameworks surrounding generative AI use in biotech are still evolving, with regulatory bodies grappling with issues surrounding data privacy, ethical considerations, and validating AI-driven solutions. Collaboration among regulatory agencies, researchers, and industry experts is vital in creating guidelines and standards to promote responsible and efficient use of generative AI in biotech.
Contact us
Contact Person:ย Mr. Lawrence John
Marketresearch.Biz
Tel:ย +1 (347) 796-4335
Send Email:ย [email protected]
Content has been published via 11press. for more details please contact at [email protected]

The team behind market.us, marketresearch.biz, market.biz and more. Our purpose is to keep our customers ahead of the game with regard to the markets. They may fluctuate up or down, but we will help you to stay ahead of the curve in these market fluctuations. Our consistent growth and ability to deliver in-depth analyses and market insight has engaged genuine market players. They have faith in us to offer the data and information they require to make balanced and decisive marketing decisions.