Generative AI in Drug Discovery Market Hit USD 1129 Mn by 2032
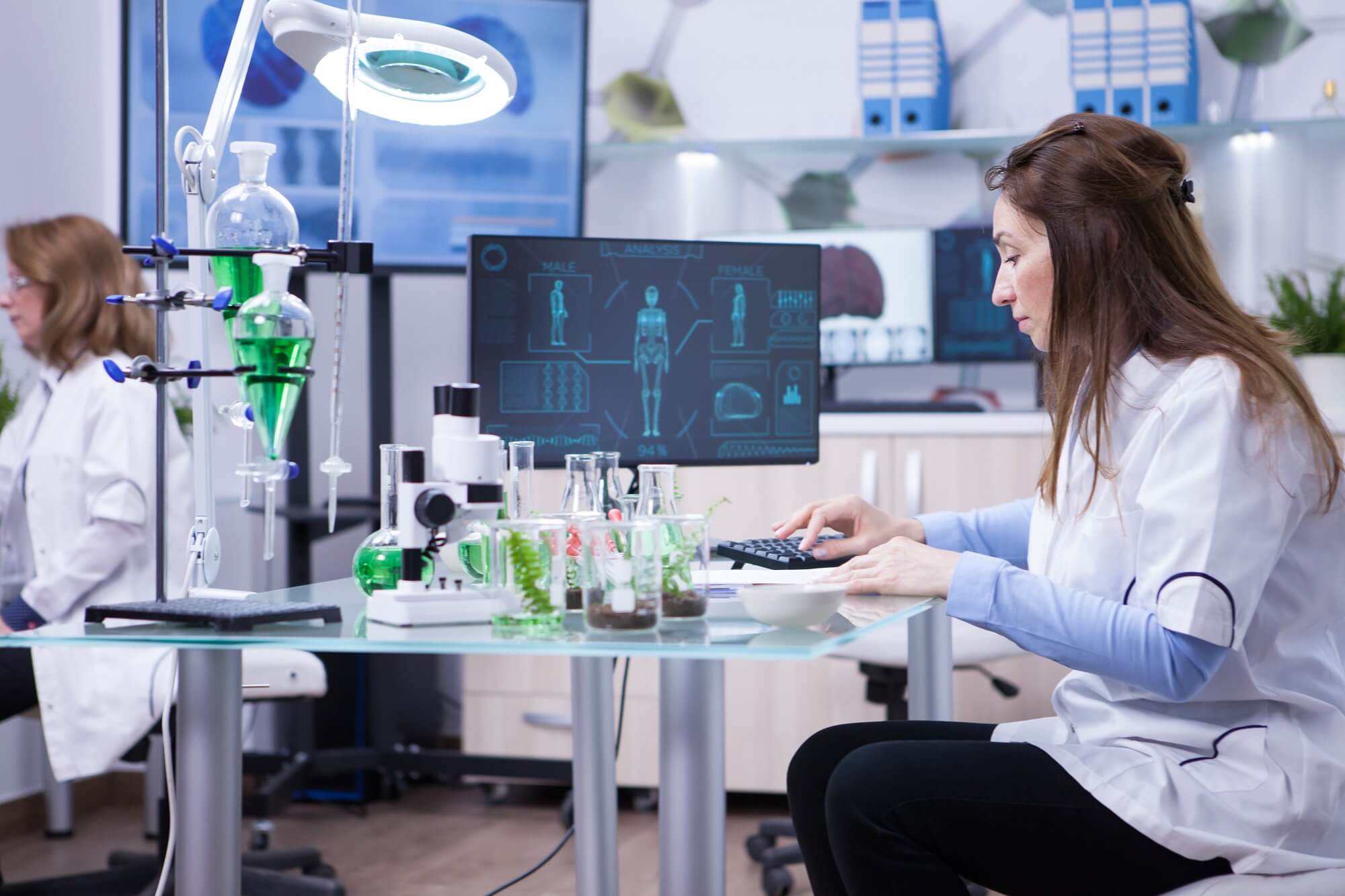
Page Contents
Market Overview
Published Via 11Press : Generative AI in Drug Discovery Market size is expected to be worth around USD 1129 Mn by 2032 from USD 109 Mn in 2022, growing at a CAGR of 27.1% during the forecast period from 2023 to 2032.
Generative AI has become an unparalleled force in drug discovery, revolutionizing how new medications are developed. Through advanced techniques such as GANs and RNNs, generative AI allows for the generation of novel molecules, prediction of their properties and optimization of drug candidates – leading to an enormous rise in its market penetration.
One factor fuelling market growth is an ever-increasing need for new drugs to address unmet medical needs. Generative AI allows researchers to explore vast chemical spaces and design molecules with specific properties, increasing their chances of discovering novel therapies. Generative AI also increases efficiency during drug discovery processes by rapidly producing molecules with desired characteristics that increase drug discovery efficiency.
Cost savings and improved efficiency also play an integral part in expanding the market. Traditional methods for drug discovery are both time-consuming, and expensive, and often yield high failure rates; Generative AI algorithms accelerate lead identification and optimization, significantly decreasing the time and costs associated with developing new drugs. Researchers who employ AI-powered approaches can focus their efforts on molecules with greater chances of success, streamlining drug development.
Big data and advances in computational power have also played an integral role in fuelling the growth of generative AI in drug discovery market. Large datasets like chemical libraries, biological assays and clinical information can all be quickly processed using these AI algorithms for analysis allowing researchers to derive meaningful insights, predict molecular properties more accurately, and quickly identify potential drug candidates more quickly than before.
Collaborations and partnerships among pharmaceutical companies, research institutions and AI startups have played a crucial role in driving forward the use of generative AI for drug discovery. By combining domain expertise with AI capabilities, these collaborations foster innovation and facilitate drug candidate identification – creating a synergy that accelerates new medication's development.
Regulatory bodies have also recognized the promise of generative AI technologies for drug discovery and have issued guidelines to integrate them into drug development processes more seamlessly. Such support from regulatory authorities further spurs the adoption of such technologies within pharmaceutical industries and leads to market expansion.
Request Sample Copy of Generative AI In Drug Discovery Market Report at: https://marketresearch.biz/report/generative-ai-in-drug-discovery-market/request-sample/
Key Takeaways
- Accelerated Drug Discovery: Generative AI rapidly generates and evaluates virtual compounds, speeding up the search for potential drug candidates.
- De Novo Design: AI-driven algorithms enable the creation of new molecules with desired properties, expanding the chemical space for drug discovery.
- Target-specific Optimization: AI optimizes drug candidates based on specific target interactions, enhancing their efficacy and reducing side effects.
- Cost Reduction: Generative AI reduces costs by minimizing the need for extensive laboratory experiments through computational modeling and prediction.
- Improved Efficiency: AI streamlines the drug discovery process, allowing researchers to focus on the most promising candidates and allocate resources effectively.
- Enhanced Target Identification: Generative AI analyzes vast biological data to identify and prioritize drug targets, improving the selection of viable options.
- Potential for Personalized Medicine: AI-driven drug discovery enables tailored treatments by considering individual patient characteristics, optimizing treatment efficacy and patient outcomes.
Regional Snapshot
North America has emerged as a significant center for AI-powered drug discovery activities, with the United States leading the pack. There is a vibrant industry comprised of pharmaceutical companies, biotech startups and research institutions that actively use AI technologies in their drug discovery initiatives. A combination of substantial funding and supportive regulatory conditions have contributed to market expansion across North America; major cities like Boston, San Francisco and San Diego have become hotbeds of such endeavors.
Europe has witnessed significant progress in generative AI drug discovery. Countries like the UK, Germany and Switzerland have witnessed an explosion in startups and academic collaborations dedicated to using AI techniques to accelerate drug discovery processes. London, Cambridge and Basel are key European cities driving innovation in this space.
Asia-Pacific countries are rapidly adopting generative AI to drug discovery, with China, Japan and South Korea making significant investments in AI research and development, including drug discovery applications. China, Japan and South Korea all possess robust pharmaceutical industries which leverage AI technologies to enhance their drug discovery pipelines. India and Singapore have also shown progress with active collaborations between academia, industry and government agencies in this field.
Latin America, the Middle East and Africa are also seeing progress with respect to AI-powered drug discovery in terms of pace of development. Over time these regions are slowly realizing the power of these AI technologies for improving pharmaceutical industry processes; initiatives and partnerships have already begun in some cases to leverage generative AI for drug discovery purposes and enhance healthcare outcomes.
Drivers
Increased Need for New and Effective Drugs: With cancer, infectious disease, neurological disorder and rare genetic conditions gaining in prevalence every day, new effective drugs must constantly be discovered and developed to combat them. Generative AI offers the potential to accelerate drug discovery processes by creating large numbers of novel molecules which can then be tested for therapeutic potential.
Rising Cost and Time Constraints: Drug development can be an expensive, time-consuming, and complex endeavor, taking years of traditional methods of drug discovery to produce results. Generative AI could shorten this process significantly by rapidly creating large libraries of virtual compounds which could then be computationally screened, saving resources while speeding up the identification of candidates for further testing.
Advancements in Computational Power: With exponential advances in computational power comes an explosion in artificial intelligence (AI) applications across numerous areas, including drug discovery. Generative AI algorithms can use this computational power to rapidly generate and analyze vast numbers of molecular structures while optimizing for specific properties like binding affinity, selectivity and bioavailability.
Large-Scale Molecular Databases: The advent of public and proprietary molecular databases has greatly expanded the capabilities of generative AI in drug discovery. These databases contain a wealth of chemical data that AI algorithms can utilize to generate novel molecules with desirable properties.
Integrating Machine Learning and Deep Learning Techniques: Integrating machine learning and deep learning techniques has become a cornerstone of generative AI in drug discovery, enabling AI models to learn from massive amounts of data, identify patterns, and produce novel molecules with desired properties based on learned patterns or rules.
Collaboration Between AI Companies and Pharmaceutical Industry: Recently, AI companies that specialize in generative AI have witnessed increasing collaboration with pharmaceutical firms to leverage AI algorithms and data analysis expertise for use in drug discovery efforts. Pharmaceutical firms partner with AI providers so as to harness the power of generative AI.
Support and Acceptance by Regulators: Regulatory agencies such as the Food and Drug Administration (FDA) have acknowledged AI's potential use in drug discovery by offering guidance for the incorporation of AI technologies into drug development processes. Such acceptance by regulatory bodies helps foster adoption of generative AI into drug discovery.
Take a look at the PDF sample of this report: https://marketresearch.biz/report/generative-ai-in-drug-discovery-market/request-sample/
Restraints
Limitations in Validation and Interpretability: Drug discovery AI models often lack adequate validation and interpretability. While AI-generated molecules may exhibit desirable properties in silico, translating their findings to real-world efficacy and safety can be challenging. Validation through preclinical and clinical studies must take place to establish potential drug candidates as effective and safe; understanding AI reasoning behind AI-generated molecules can make understanding their underlying mechanisms and making informed decisions more challenging than it needs to be.
Quality and Reliability Issues: Generative AI-generated molecules remain of concern due to their quality and reliability issues. Though AI algorithms can produce large numbers of molecules, there remains the risk that some may be chemically unstable, toxic, or have low chances of success in clinical trials. Due to a lack of robustness and generalizability within AI models, false positives or false negatives may occur resulting in inefficient resource allocation or wasted efforts during experimental validations.
Regulatory and Ethical Challenges: The regulatory landscape surrounding AI-generated molecules used in drug discovery is still evolving, as regulatory agencies often require extensive safety and efficacy data before creating the regulatory framework for these AI-produced compounds. Ethical implications must also be carefully considered such as fairness, biases, patient privacy security, etc. Successfully overcoming these hurdles requires collaboration among AI developers, pharmaceutical companies and regulatory authorities in creating guidelines and standards.
Opportunities
Generative AI Can Accelerate Drug Discovery Process: Generative AI has the potential to dramatically speed up drug discovery processes by creating large libraries of virtual compounds which can be screened computationally, which in turn enables pharmaceutical companies to identify potential drug candidates more quickly – cutting both time and cost associated with traditional methods. Being able to generate and evaluate large amounts of molecules simultaneously allows a more effective exploration of chemical space which may result in the discovery of novel and effective therapeutics.
Targeting Undruggable Proteins: Generative AI can aid in targeting previously considered “undruggable” proteins, as many diseases involve dysfunctioning proteins that were difficult to target with small-molecule drugs. Generative AI algorithms can generate molecules designed specifically to interact with these proteins, providing new possibilities for drug discovery that expand potential target spaces and can ultimately result in therapies for diseases that were once difficult to treat.
Drug Repurposing and Combination Therapy: Generative AI can assist in finding new uses for existing drugs through the process of drug repurposing. AI algorithms can leverage drug molecular structures and properties to develop insights into potential therapeutic uses beyond their original indication. Repurposing drugs can significantly decrease time and costs associated with clinical development as their safety profiles have already been established. Generative AI can also aid the design of combination therapies, in which multiple drugs are combined together to increase therapeutic efficacy. Artificial intelligence algorithms can generate novel combinations of drugs and optimize interactions, leading to synergistic effects and improved treatment outcomes.
Collaboration and Data Sharing: Generative AI has provided pharmaceutical companies, AI developers, and academic institutions an incentive for increased collaboration and data sharing amongst themselves and each other. Sharing molecular databases, research findings, AI models can lead to advances in drug discovery as a whole and foster innovation; while collaborative efforts may help address data quality challenges such as diversity or validation thus leading to more reliable AI models.
Challenges
Data Quality and Availability: Both quality and availability of data are essential in training generative AI models for drug discovery. Unfortunately, finding high-quality, well-curated, diverse training data may be limited – this requires conducting extensive experiments which may cost time and money to obtain accurate molecular data for training AI models; furthermore, ideally, training data sets must represent desired properties of molecules being created – creating another challenge when selecting suitable training data sets for AI training purposes.
Interpretability and Explainability: Generative AI models often lack interpretability and explainability, making it challenging for researchers to comprehend the underlying logic behind their generated molecules. Interpreting AI algorithms' reasoning and decision-making process is vital in order for researchers to have faith in their output molecules and make informed decisions regarding potential candidates as drug candidates. Creating methods to enhance interpretability and explainability in drug discovery remains an ongoing research and development challenge that needs further exploration and research.
Experimental Validation and Cost: Although generative AI can generate large numbers of potential drug candidates, experimental validation is still essential to verify their efficacy, safety and pharmacokinetic properties. Experimental validation usually entails preclinical or clinical studies that require time-intensive, resource-intensive and expensive studies; prioritizing and selecting only promising molecules generated for experimental validation from among all those generated is also a major challenge during drug discovery; efficient yet cost-effective experimental validation strategies must also be devised so as to maximize resource use efficiency.
Regulation and Ethical Considerations: Generative AI's application in drug discovery raises both regulatory and ethical considerations. At present, regulatory agencies are still formulating policies regarding their evaluation and approval of AI-generated molecules; without established pathways or sufficient safety and efficacy data for approval, this presents challenges in bringing them to market. Furthermore, ethical issues related to data privacy, biased training data or potential risks arising from AI use pose additional concerns; to ensure acceptance and adoption generative AI must adhere to ethical standards while remaining transparent, fairness and ethical compliance are crucial aspects of adoption and adoption within drug discovery applications.
For any inquiries, Speak to our expert at: https://marketresearch.biz/report/generative-ai-in-drug-discovery-market/#inquiry
Market Segmentation
Based on Technology
- Machine learning
- Reinforcement learning
- Deep learning
- Molecular docking
- Quantum computing
Based on End-User
- pharmaceutical and biotechnology companies
- academic and research institutions
- contract research organizations (CROs)
- Other End-Users
Key Players
- Insilico Medicine
- Atomwise Inc.
- BenevolentAI
- XtalPi Inc
- Numerate Inc
- Cyclica Inc
- BioSymetrics
- Other Key Players
Report Scope
Report Attribute | Details |
Market size value in 2022 | USD 109 Mn |
Revenue forecast by 2032 | USD 1129 Mn |
Growth Rate | CAGR Of 27.1% |
Regions Covered | North America, Europe, Asia Pacific, Latin America, and Middle East & Africa, and Rest of the World |
Historical Years | 2017-2022 |
Base Year | 2022 |
Estimated Year | 2023 |
Short-Term Projection Year | 2028 |
Long-Term Projected Year | 2032 |
Request Customization Of The Report: https://marketresearch.biz/report/generative-ai-in-drug-discovery-market/#request-for-customization
Recent Developments
In September 2020 Exscientia, an AI-driven drug discovery company, announced a three-year strategic collaboration with Bayer. This agreement focused on Exscientia using its AI platform to discover small molecule drug candidates for cardiovascular and oncology indications using its platform; further payments may include an upfront payment, potential milestone payments and royalties on any successful programs.
In March 2020 Atomwise, an AI-driven drug discovery company, announced a research collaboration agreement with Hansoh Pharmaceutical – one of China's premier pharmaceutical firms – to identify and develop potential drug candidates using Atomwise's AI technology against several undisclosed targets. Financial terms included research funding as well as potential milestone payments.
In 2019, Insilico Medicine, an AI-driven drug discovery company, and GSK announced a research collaboration that leveraged Insilico Medicine's AI technology to uncover novel targets and potential therapies across multiple disease areas. Financial terms included upfront payments, research funding and potential milestone payments.
In 2018, Recursion Pharmaceuticals, an AI and automation-powered drug discovery company, announced a multi-year research collaboration with Sanofi to identify potential candidates for rare genetic diseases using AI/automation techniques. The agreement includes an upfront payment, research funding, and milestone payments contingent on the successful development/commercialization of compounds.
FAQ
What is Generative AI Used for in Drug Discovery?
Generative AI in drug discovery refers to the application of artificial intelligence algorithms in order to generate novel molecules with desired properties, using machine learning and deep learning techniques in combination to design and optimize potential drug candidates.
How does generative AI assist with drug discovery?
Generative AI algorithms in drug discovery work by learning patterns and rules from large molecular databases. They then create new molecules by combining existing chemical structures together while optimizing for specific properties using techniques such as reinforcement learning or generative adversarial networks.
What are the advantages of employing generative AI for drug discovery?
Generative AI drug discovery provides numerous advantages, such as a speedy drug discovery process, exploration of chemical space, identification of novel drug candidates, repurposing existing medications and personalized medicine approaches. Generative AI can significantly speed up the identification of potential therapeutics while simultaneously increasing efficiency within the drug development pipeline.
What are the applications of Generative AI in drug discovery?
Generative AI for drug discovery has multiple applications in drug discovery, from target identification and lead generation through hit-to-lead optimization, de novo drug design, drug repurposing and combination therapy design. Generative AI provides invaluable assistance in the discovery and development of new medications for various diseases.
What are the challenges associated with using generative AI for drug discovery?
Frustrations associated with using generative AI for drug discovery include data quality and availability issues, interpretability/explainability of generated molecules, experimental validation cost issues, regulatory concerns and ethical considerations – each must be overcome for its successful implementation in this field.
How does Generative AI complement traditional drug discovery methods?
Generative AI complements traditional drug discovery methods by efficiently exploring chemical space and creating diverse molecules for screening. Generative AI also assists researchers with the identification of novel chemical scaffolds and can speed up the lead discovery process. By combining its strengths with traditional approaches, researchers can improve both the efficiency and success rates of drug discovery efforts.
Are there any examples of the successful use of Generative AI in drug discovery?
Yes, there have been successful examples of generative AI being utilized for drug discovery. For instance, these algorithms have been utilized to discover potential drug candidates for diseases such as malaria, cancer and COVID-19 using AI-generated molecules which then progressed into preclinical and clinical studies, showing its promise.
How Does Generative AI Affect Drug Development Time and Costs?
Generative AI holds great promise to shorten drug development timelines and costs significantly. By rapidly creating and screening large libraries of virtual compounds, researchers can identify potential drug candidates that meet clinical trials' criteria more quickly – saving both time and resources by prioritizing molecules with higher chances of success in order to expedite drug development processes more efficiently.
What are the potential uses for Generative AI in drug discovery?
Future prospects of generative AI for drug discovery look promising. As AI algorithms continue to advance, we can anticipate greater accuracy and efficiency in producing molecules with desired properties. Integrating generative AI with other technologies such as high-throughput screening or robotic automation will enhance this phase of drug discovery even further.
How can companies and researchers utilize generative AI for drug discovery?
Companies and researchers can take advantage of generative AI in drug discovery by partnering with AI companies, investing in AI technology infrastructure and developing in-house expertise in AI and machine learning. Partnerships between pharmaceutical companies and AI startups may further speed the adoption and implementation of this innovative tool for drug discovery workflows.
Contact us
Contact Person: Mr. Lawrence John
Marketresearch.Biz
Tel: +1 (347) 796-4335
Send Email: [email protected]
Content has been published via 11press. for more details please contact at [email protected]

The team behind market.us, marketresearch.biz, market.biz and more. Our purpose is to keep our customers ahead of the game with regard to the markets. They may fluctuate up or down, but we will help you to stay ahead of the curve in these market fluctuations. Our consistent growth and ability to deliver in-depth analyses and market insight has engaged genuine market players. They have faith in us to offer the data and information they require to make balanced and decisive marketing decisions.