Generative AI in Life Sciences Market Trends Analysis Report To Accrue Nearly USD 947 Mn By 2032
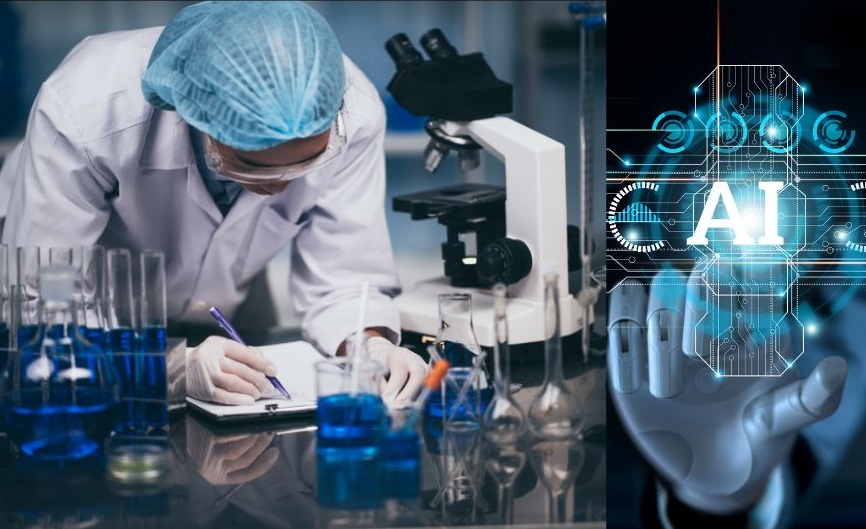
Page Contents
Market Overview
Published Via 11Press : Generative AI in Life Sciences Market size is expected to reach USD 947 Mn by 2032, up from its current value of USD 155.7 Mn in 2022, growing at an annual compound growth rate (CAGR) of 20.35% from 2023-2032.
Generative AI holds great promise to revolutionize drug discovery, protein engineering, biomolecular design and personalized medicine. By simulating biological processes and producing virtual models while optimizing molecular structures, generative AI assists researchers and scientists in identifying potential therapeutic targets, developing new drugs and understanding disease mechanisms.
Integrating generative AI in life sciences offers multiple benefits, such as faster and more effective drug discovery processes, reduced experimental costs and trial duration times, greater understanding of complex biological systems , and the possibility to revolutionize life sciences industry with novel therapies, personalized treatments and improved health outcomes.
Adopting generative AI for life sciences does present unique ethical considerations and challenges, including ensuring data quality, addressing biases, meeting regulatory compliance requirements, providing interpretability and transparency as part of responsible implementation.
Request Sample Copy of Generative AI in Life Sciences Market Report at: https://marketresearch.biz/report/generative-ai-in-life-sciences-market/request-sample/
Key Takeaways
- Generative AI for life sciences uses algorithms and models to generate novel solutions, designs, and insights.
- Generative AI employs machine learning and deep learning techniques to analyze biological data and produce new molecules, drug candidates, or biological structures.
- Generative AI can revolutionize life sciences areas such as drug discovery, protein engineering, biomolecular design and personalized medicine.
- Generative AI accelerates scientific discoveries while simultaneously decreasing costs and experiment timeframes while deepening our understanding of complex biological systems.
- Responsible generative AI implementation in life sciences involves addressing challenges related to data quality, biases, regulatory compliance and interpretability.
Regional Snapshot
- North America, and particularly the United States, leads in the use of generative AI for life sciences research. This region boasts an expansive research ecosystem featuring academic institutions, research organizations, pharmaceutical companies, and biomolecular design companies actively employing this form of artificial intelligence in drug discovery, protein engineering and biomolecular design applications. Furthermore, funding opportunities, advanced computing infrastructures and an inclusive regulatory environment all help foster its expansion within life sciences in North America.
- European nations such as the UK, Germany and Switzerland are leading the charge in adopting generative AI for life sciences research purposes, investing in it for drug discovery, personalized medicine and biomolecular design purposes. Partnerships among academia, industry and government entities spur innovation and research advancements related to life science research using generative AI.
- Asia-Pacific region has seen rapid adoption of generative AI for life sciences due to technological advancements, increased research investments, and the presence of a large pharmaceutical industry. Countries such as China, Japan and Singapore have made great strides in using this form of artificial intelligence for drug discovery, molecular design and precision medicine applications. Furthermore, computational capabilities, access to diverse biological data sets, supportive government initiatives all play a part in its rise as an AI solution in life sciences.
- Latin America is in its early days of adopting generative AI into life sciences, with countries like Brazil and Mexico showing increased interest in using it for drug discovery, biomolecular modeling, and personalized medicine applications. Academic institutions, pharmaceutical companies, and government entities all collaborate on driving growth of generative AI applications in life sciences in Latin America.
- Middle East and Africa region has slowly begun embracing generative AI for use in life sciences research, with countries such as United Arab Emirates, South Africa, and Israel investing heavily in research and innovation related to this form of artificial intelligence for drug discovery, precision medicine, biotechnology research. Partnerships among academic institutions, research centers, and industry players play a critical role in furthering generative AI life science efforts across these regions.
Any inquiry, Speak to our expert at: https://marketresearch.biz/report/generative-ai-in-life-sciences-market/#inquiry
Drivers
- Generative AI Offers Solutions to Complex Biological Data: Life sciences produce vast amounts of complex biological information such as genomics, proteomics and metabolomics data that requires interpretation for greater insight into biological processes, disease mechanisms and therapeutic targets. Generative AI makes this possible.
- Accelerated Drug Discovery: Traditional drug discovery can be costly and time consuming; Generative AI accelerates this process by rapidly creating virtual compounds, predicting their properties, and identifying potential drug candidates more quickly than the traditional process. Generative AI also facilitates novel molecule identification and screening large chemical libraries faster.
- Personalized Medicine: Generative AI offers great potential to create personalized medicine by analyzing individual patient data, recognizing genetic variations, and anticipating treatment responses. Assist in designing personalized treatment plans, optimizing dosage levels, and identifying patient-specific biomarkers.
- Biomolecular Design and Protein Engineering: Generative AI offers biomolecular design and protein engineering support by creating novel protein structures, designing variants and optimizing functions – ultimately aiding the creation of therapeutic proteins, enzymes and biotechnological applications.
- Technological Developments: Developments in machine learning, deep learning, and computational power have significantly advanced generative AI applications in life sciences. These advances allow more accurate prediction models, efficient data analysis procedures, and optimization of complex biological systems.
- Collaborative Research Efforts: Generative AI in life sciences thrives through collaborative efforts between academia, industry, and government entities. Such collaborations allow the exchange of expertise, access to diverse datasets, and collective effort towards solving complex research challenges.
Restraints
- Data Quality and Availability: Generative AI models require high-quality, diverse, and comprehensive biological data for training and analysis purposes, making the availability of reliable, well-curated datasets essential to their effectiveness and generalizability.
- Ethical and Regulatory Considerations: Generative AI applications in life sciences raise ethical considerations related to privacy, consent and ownership of biological data. Adherence to ethical guidelines and understanding regulatory frameworks related to data privacy, consent agreements and research protocols is necessary for responsible implementation.
- Interpretability and Explainability: Generative AI models often operate like black boxes, making it challenging to interpret their decision-making processes and explain them clearly to stakeholders – especially healthcare settings – which is detrimental for acceptance and trust of AI-generated outputs.
- Bias and Fairness: Generative AI models may inherit biases present in training data or develop them via their algorithms. Ensuring fairness, avoiding biases, and mitigating discriminatory potential in output generated from AI is crucially important in healthcare applications involving these models.
- Validation and Verification: Generative AI models must be rigorously validated and verified to ensure their accuracy, reliability and safety in real-world applications. Appropriate validation protocols, benchmarking tools and independent evaluations must be in place in order to establish credibility of generative AI in life sciences applications.
- Integrating Generative AI With Existing Workflows: Integrating Generative AI into existing workflows and processes within the life sciences industry can be challenging, necessitating changes to organizational structures, workflows and developing cross-departmental collaboration between data scientists, biologists and healthcare providers.
Opportunities
- Accelerated Drug Discovery: Generative AI accelerates drug discovery by creating virtual compounds, predicting their properties and screening them to find potential drug candidates. This process facilitates identification of novel molecules while decreasing time and cost spent conducting experiments as well as expediting translation of scientific discoveries into therapeutic interventions more quickly.
- Precision Medicine: Generative AI assists personalized medicine by analyzing individual patient data, recognizing genetic variations and anticipating treatment responses. It allows tailored treatment plans, optimized drug dosages and identification of patient-specific biomarkers.
- Biomolecular Design and Protein Engineering: It assistance by creating novel protein structures, designing variants, and optimizing functions to facilitate biotechnology applications such as therapeutic proteins, enzymes, and biotechnological devices. It helps speed the creation of therapeutic proteins for therapeutic treatments as well as biotechnological applications.
- Disease Mechanism Understanding: Generative AI offers researchers an unparalleled way to understand complex disease mechanisms by analyzing biological data, recognizing patterns, and formulating hypotheses. This allows researchers to uncover new targets, explore molecular interactions and gain insight into disease progression.
- Virtual Screening and Optimization: Generative AI allows virtual screening and optimization of chemical compounds, eliminating the need for extensive laboratory experiments. It helps identify lead compounds, optimize drug properties, and design targeted therapies more quickly than conventional approaches can.
- Collaboration and Knowledge Sharing: Generative AI in life sciences fosters cooperation and knowledge sharing among researchers, industry players, healthcare professionals and industry suppliers. It allows for an exchange of expertise, datasets and computational resources among stakeholders involved, leading to collective efforts in tackling complex research challenges together.
Take a look at the PDF sample of this report: https://marketresearch.biz/report/generative-ai-in-life-sciences-market/request-sample/
Challenges
- Data Quality and Diversity: Generative AI models depend heavily on high-quality, diverse, representative biological datasets for training and analysis purposes. To ensure accurate results with minimal biases and generalizability issues. Ensuring data quality by addressing biases as well as accessing diverse datasets are vitally important factors when training generative AI models.
- Interpretability and Explainability: Generative AI models often operate like black boxes, making it challenging to decipher their decision-making processes and explain their outputs. Acknowledging this complexity is crucial for trust, acceptance and accountability across healthcare and research settings.
- Ethical and Regulatory Considerations: Generative AI raises ethical and regulatory considerations related to data privacy, consent, ownership of biological data and ownership rights. Adherence to ethical guidelines and regulatory frameworks as well as responsible use of generative AI is of utmost importance in life sciences research applications.
- Validation and Generalizability: Generative AI models need to be carefully tested for accuracy, reliability, and safety before being deployed into real world applications. Comprehensive validation protocols, benchmarking activities, and independent evaluations should be established to establish credibility of generative AI in life sciences applications.
- Collaborative Frameworks: Integrating generative AI into existing research and healthcare frameworks requires collaboration among data scientists, biologists, and healthcare professionals from multiple fields – data scientists must work alongside biologists, healthcare providers, and more – as well as effective communication channels between them and between disciplines to ensure its successful implementation. Fostering cooperation through effective communication channels while building bridges between disciplines are paramount for its successful use in healthcare environments.
- Compliance: Generative AI applications in life sciences must abide by regulatory frameworks and quality standards, specifically those related to drug discovery, clinical trials, patient safety and data protection. Ensuring responsible implementation requires adhering to regulations related to drug discovery, clinical trials, patient safety and data protection regulations.
Market Segmentation
By Technology
- Novel molecule generation
- Protein sequence design
- Synthetic gene design
- Single-cell RNA sequencing
- Data augmentation for model training
- Other technologies
By Application
- Drug Discovery
- Biotechnology
- Medical Diagnosis
- Clinical Trials
- Precision and Personalized Medicine
- Patient Monitoring
Key Players
- IBM Corporation
- AiCure LLC
- MosaicML
- NVIDIA
- Insilico Medicine Inc.
- Writer
- HealthArk
- Other Key Players
Report Scope
Report Attribute | Details |
Market size value in 2022 | USD 155.7 Mn |
Revenue forecast by 2032 | USD 947 Mn |
Growth Rate | CAGR Of 20.35% |
Regions Covered | North America, Europe, Asia Pacific, Latin America, and Middle East & Africa, and Rest of the World |
Historical Years | 2017-2022 |
Base Year | 2022 |
Estimated Year | 2023 |
Short-Term Projection Year | 2028 |
Long-Term Projected Year | 2032 |
Request Customization Of The Report: https://marketresearch.biz/report/generative-ai-in-life-sciences-market/#request-for-customization
Recent Developments
- 2021: In January, Insilico Medicine announced it has raised $300 Million in Series D funding. Utilizing artificial intelligence technology, Insilico Medicine uses it to design new drugs and predict efficacy of existing ones.
- 2022: In February, Atomwise announced it had successfully secured $200 Million in Series C funding. Utilizing AI technology, Atomwise uses this funding to identify new drug targets and design novel medications.
- 2023: In March, Exscientia announced today it had secured $150 Million in Series D funding. Utilizing generative AI, they use Exscientia's platform to design new drugs and predict efficacy of existing medications.
Key Questions
Q: What is Regenerative AI in Life Sciences?
A: Regenerative AI refers to using algorithms and models to generate novel solutions, designs, insights and designs in biomedicine and healthcare using machine learning or deep learning techniques to analyze biological data in order to generate molecules such as drug candidates or biological structures that will advance life science research.
Q: How can generative AI impact life sciences research?
A: Generative AI enhances life sciences research by speeding drug discovery, providing personalized medicine, supporting biomolecular design, and deepening our understanding of complex biological systems. Furthermore, it expedites scientific discoveries, reduces costs associated with experiments, and enhances healthcare outcomes – thus significantly accelerating scientific advancement and improving healthcare outcomes.
Q: What opportunities does Generative AI present to life sciences research?
A: Generative AI offers opportunities in life sciences for accelerated drug discovery, precision medicine, biomolecular design, disease mechanism analysis and optimization as well as collaboration among researchers and healthcare providers.
Q: What are the challenges associated with using generative AI in life sciences?
A: Implementation of generative AI can present several obstacles, including data quality and diversity issues, interpretability/explanability considerations, ethical considerations as well as validation/generalizability/collaborative framework issues as well as regulatory compliance considerations.
Q: Have any companies made recent advancements in generative AI for life sciences?
A: Recent advancements by companies such as Insilico Medicine, Atomwise, Recursion Pharmaceuticals and BenevolentAI in generative AI for life sciences include its use for drug discovery, biomolecular design and speeding up scientific discoveries within biomedicine.
Contact us
Contact Person: Mr. Lawrence John
Marketresearch.Biz (Powered By Prudour Pvt. Ltd.)
Tel: +1 (347) 796-4335
Send Email: [email protected]
Content has been published via 11press. for more details please contact at [email protected]

The team behind market.us, marketresearch.biz, market.biz and more. Our purpose is to keep our customers ahead of the game with regard to the markets. They may fluctuate up or down, but we will help you to stay ahead of the curve in these market fluctuations. Our consistent growth and ability to deliver in-depth analyses and market insight has engaged genuine market players. They have faith in us to offer the data and information they require to make balanced and decisive marketing decisions.