Generative AI in Pharmaceuticals (Revolutionizing Drug Discovery)
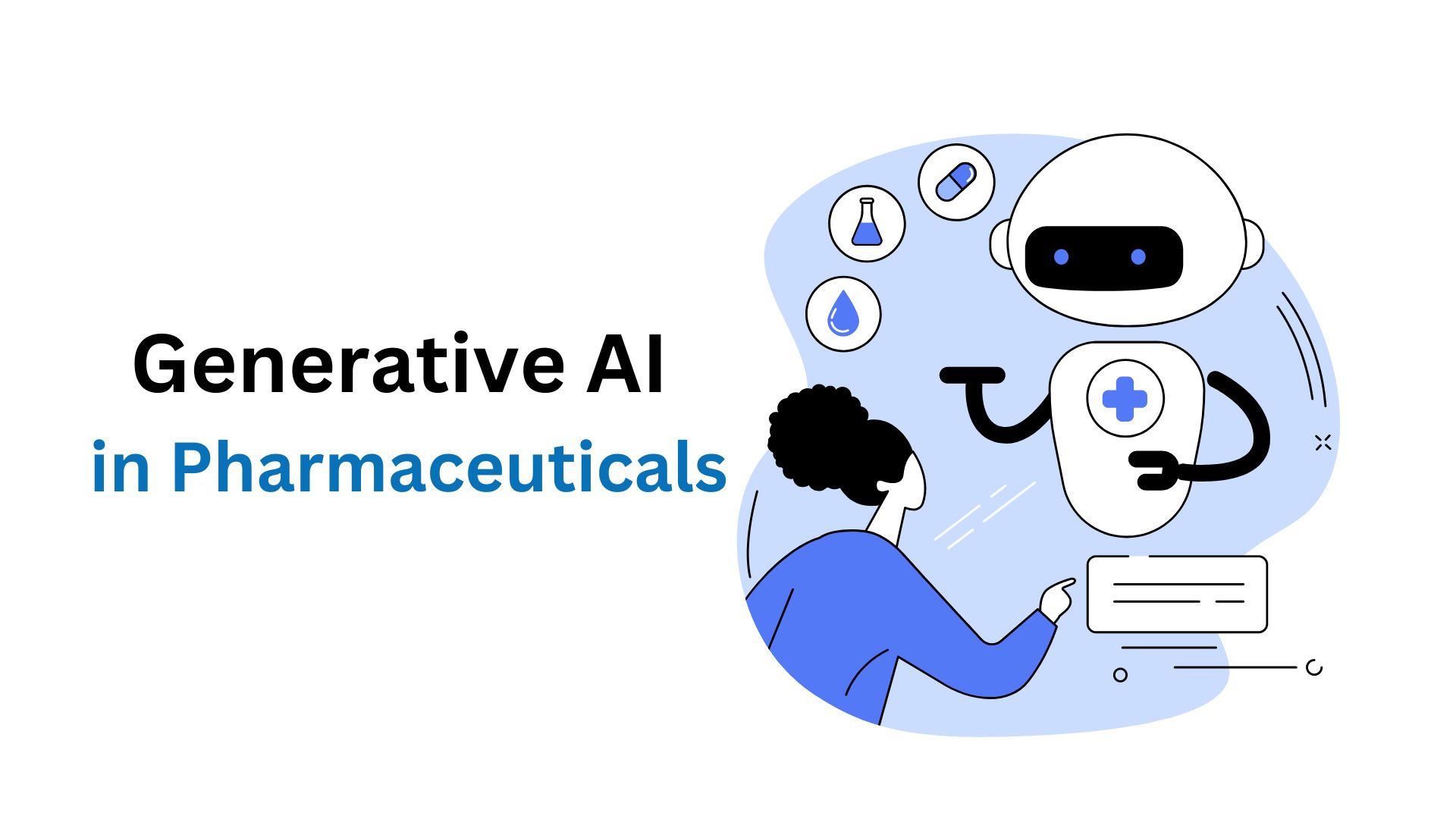
Page Contents
- Introduction to Generative AI
- What's Generative AI?
- Which kinds of Generative AI tools are utilized in the Pharmaceutical Industry?
- What are the uses of Generative AI in the Pharmaceutical field?
- How Generative AI Can Change the Pharmaceutical Industry?
- Generative AI In Drug Discovery Market and Forecast
- Challenges of Generative AI in Pharmaceuticals?
- Conclusion
Introduction to Generative AI
Artificial Intelligence (AI) is a fast-evolving technology that has been changing various industries in recent years. Whether it's healthcare, manufacturing, or other sectors, AI is changing the way we work. One industry that's adopted AI early on is the pharmaceutical industry, particularly in the discovery and creation of new drugs. This article will highlight a specific AI aspect, Generative AI, and its role in the pharmaceutical sector.
What's Generative AI?
Generative AI is a type of AI that uses mathematical rules to create new information that's similar to the original data it was taught with. The original data can be anything – pictures, written words, or even the structure of chemicals. As a part of machine learning, Generative AI uses what we call neural networks to understand patterns in the original data and create new data that resembles it. This technology has great potential in the pharmaceutical industry, especially when it comes to discovering and developing new drugs. By creating new molecules with specific features, Generative AI can speed up the drug discovery process, helping pharmaceutical companies create new medicines faster and more effectively.
Generative AI operates using a machine learning method that involves two neural networks – one that generates and another that discriminates. The generator network creates new data that imitates real-world information, while the discriminator network tries to tell the difference between the created data and the real-world data. With time, the generator network gets better at making data that's nearly indistinguishable from real-world information. Generative AI falls under a category of machine learning known as unsupervised learning, which means it can learn from data that hasn't been labelled. This is a big plus, as it allows the technology to create new data without the need for existing datasets.
Which kinds of Generative AI tools are utilized in the Pharmaceutical Industry?
There are several types of Generative AI tools that the pharmacy field uses to tackle different issues and tasks. Here are some of the commonly used Generative AI tools in the pharmacy sector:
- Generative Adversarial Networks (GANs): These tools are popular in pharmacy research for creating new drugs and generating molecules. GANs have a generator network that makes synthetic samples and a discriminator network that checks if these samples are authentic. This process leads to the creation of new molecules with the desired features.
- Recurrent Neural Networks (RNNs): RNNs are used for creating sequential data, which is useful in forming new chemical structures or improving the properties of drugs. RNNs learn the patterns in sequential data, which allows them to make new sequences that have the desired characteristics.
- Variational Autoencoders (VAEs): These tools are used in discovering and improving drugs. VAEs understand the distribution of chemical structures and can create new molecules with specific features. They are very useful in examining the chemical space and creating diverse compounds.
- Deep Reinforcement Learning: This type of learning is used in discovering and improving drugs. These algorithms learn by trying and making errors, and they can create molecules with the desired features by maximizing rewards based on pre-set objectives.
- Transformer Models: Transformer models, like the popular GPT (Generative Pre-trained Transformer) model, are used in tasks that involve processing natural language, like creating text and discovering drugs. These models can create text that makes sense and is relevant to the context, which makes them useful for creating descriptions of chemicals and text related to drugs.
It's crucial to remember that Generative AI is a field that is constantly changing. New tools and techniques are being created and adjusted to tackle the unique challenges in the pharmacy field. Which Generative AI tool to use depends on the task at hand and the results that pharmacy researchers and professionals want to achieve.
What are the uses of Generative AI in the Pharmaceutical field?
Here are some ways that Generative AI is used in the pharmaceutical industry:
-
Creating New Drugs/Drug Discovery
Creating new drugs can take a long time and involve testing millions of different compounds. Generative AI can speed this up by making new molecules that are specifically designed to deal with certain diseases or health problems. The molecules can be made to work better and be safer. They can also be created so they can be absorbed, distributed, metabolized, and excreted effectively in the body.
Generative AI can also make completely new chemical compounds that have never been made before. This could lead to new drugs that work in unique ways and could be used to treat diseases that we don't have good treatments for yet.
-
Improving Drug Candidates/Lead Optimization
When a drug candidate has been found, the next step is to make it better. This is done by changing the structure of the drug molecule to make it work better, be safer, and be absorbed, distributed, metabolized, and excreted effectively. Generative AI can help with this by making new molecules that are similar to the drug candidate but have better properties. These new molecules can then be made and tested to see how well they work and how safe they are.
-
Finding New Uses for Drugs/Drug Repurposing
Another use for Generative AI is to find new uses for drugs that already exist. Generative AI can do this by making new molecules that are similar to existing drugs but work differently. These new molecules can then be tested to see if they could be used to treat other diseases or health problems.
-
Creating Personalized Medicines
A new area of medicine is being developed that creates treatments that are specifically designed for individual patients. This is based on their genes and other things that are unique to them. Generative AI can help create these personalized drugs by making new molecules that are specifically designed for certain groups of patients. Generative AI could help make treatments work better and have fewer side effects by making new molecules that are specifically tailored to individual patients.
How Generative AI Can Change the Pharmaceutical Industry?
The pharmaceutical industry, which is subject to many rules and regulations, takes a long time and costs a lot to create new drugs. This process involves a lot of testing and approval from authorities like the FDA (Food and Drug Administration). However, generative AI can bring a big change to this process by making it faster and less costly to get a new drug to the market.
-
Drug Discovery
Generative AI can be very helpful in finding new drugs. This involves figuring out and testing possible new drug candidates to see if they are safe and effective.
Usually, to find new drugs, we have to check a lot of chemical compounds to see if they could be helpful for treating a disease. This process takes a long time and costs a lot of money. Also, many of the compounds we check end up not being useful.
Generative AI can make the process of finding new drugs faster by creating new molecules with specific properties. For example, a generative AI program could be trained to create molecules that can attach themselves to a certain protein. This could greatly reduce the time and money needed to find potential new drugs.
-
Drug Design
After finding a possible drug candidate, the next step is to create a drug that can effectively interact with the protein we want to target. This complex process involves making and testing many variations of the drug.
Generative AI can be used to create new drugs by creating molecular structures that are designed for certain properties. For example, a generative AI program could be trained to create molecules that are designed to be strong, selective, and easily taken up by the body.
-
Clinical Trials
Testing new drugs in clinical trials is a very important part of creating new drugs. These tests are used to check if new drugs are safe and effective before they can be approved for use.
Generative AI can make clinical trials more efficient by figuring out which groups of patients are likely to respond well to a certain treatment. For example, a generative AI program could be trained to find genetic markers that can predict if a patient will respond well to a certain drug.
Generative AI In Drug Discovery Market and Forecast
The Generative AI in Drug Discovery Market is projected to reach a value of approximately USD 1129 million by 2032, growing at a compound annual growth rate (CAGR) of 27.1% during the forecast period from 2023 to 2032. This information is based on a report by MarketResearch.Biz.
Driving Factor
One of the main factors driving the growth of this market is the increasing demand for efficient and cost-effective drug discovery processes. Generative AI offers the potential to maximize the effectiveness and efficiency of drug discovery, leading to the development of more successful and affordable drugs.
Restraining Factor
However, there are some challenges that may impede the growth of the Generative AI in Drug Discovery Market. These include a shortage of skilled professionals who are capable of effectively utilizing generative AI technologies, as well as the high costs associated with implementing and maintaining these advanced systems.
Growth Opportunity
The adoption of generative AI in drug discovery presents a significant opportunity to minimize both the time and cost involved in the drug development process. By leveraging AI algorithms and technologies, researchers and pharmaceutical companies can accelerate the discovery and design of new drugs, leading to faster and more cost-effective development cycles.
Latest Trends
Deep learning algorithms are being extensively utilized in the field of generative AI in drug discovery. These algorithms have the capability to analyze and interpret complex datasets, enabling more accurate predictions and insights into drug discovery processes. The increased use of deep learning algorithms is expected to further enhance the efficiency and effectiveness of generative AI in drug discovery.
Regional Analysis
In 2022, North America accounted for the largest revenue share in the Generative AI in Drug Discovery Market. This can be attributed to the presence of well-established pharmaceutical companies, advanced research infrastructure, and significant investments in AI technologies within the region.
Key Players
Some of the key players in the Generative AI in Drug Discovery Market include Insilico Medicine, Atomwise Inc., BenevolentAI, XtalPi Inc., Numerate Inc., Cyclica Inc., BioSymetrics, and other prominent companies operating in this space. These companies are actively involved in developing and implementing generative AI solutions for drug discovery purposes, driving innovation and advancement in the field.
Challenges of Generative AI in Pharmaceuticals?
- Quality of Data: A big issue is the quality of the data used to train the Generative AI. If the data is not good, the AI might make wrong models and predictions.
- Ethical Issues: There are also worries about how Generative AI can be misused. For example, it could be used to make new drugs without doing the proper tests. Or, it could be used to make drugs that only work for certain people, which might make it harder for everyone to get good healthcare.
- Understanding the AI: Generative AI often works in a way that's hard to understand and explain. This can be a problem in the drug industry, where it's important to be able to explain how things work for the sake of getting approval and meeting ethical standards.
- Ethics and Privacy: There are also worries about patient privacy and data protection when using Generative AI. There might also be biases in the data used to train the AI. It's very important to think about these ethical issues and use AI responsibly.
- Rules and Regulations: The drug industry has to follow a lot of rules. Any new technology, like Generative AI, has to be approved by the authorities. This can take a lot of time and money.
- Testing the AI: Before using Generative AI for important tasks in the drug industry, it has to be tested to make sure it's accurate and reliable. This can take a lot of time and be quite complicated.
- Working Together: To use Generative AI in the drug industry, AI experts, drug researchers, and other specialists need to work together. It might be difficult to bring together all these different areas of expertise.
- Ownership: There are questions about who owns the rights to drugs made using Generative AI.
- Using AI with Current Systems: It might be hard to get Generative AI to work with the systems already being used in the drug industry. Drug companies might also need to buy new equipment and software to use Generative AI.
- Expertise: Using Generative AI requires a lot of technical knowledge. Companies might need to hire more people with skills in machine learning, deep learning, and data science to use this technology properly.
- Cost: Generative AI can be costly, especially in the early stages of development and use. Companies have to think carefully about whether it's worth it to invest in this technology.
Even though Generative AI has a lot of potential for the drug industry, there are many challenges. Drug companies need to think carefully about these challenges and make sure they have the right resources and skills to use this technology properly. They will need to bring together experts in AI, drug research, rules and regulations, and data privacy to overcome these challenges. This could lead to big advancements in finding new drugs, personalized medicine, and better health outcomes in the drug industry.
Conclusion
Generative AI can change the pharmaceutical industry by making it faster and cheaper to create new drugs. It helps speed up the process of finding and designing drugs, as well as making clinical trials more efficient. But before generative AI can be used widely in the pharmaceutical industry, there are some obstacles that need to be overcome. We need bigger and more varied sets of data, and we also need reliable ways to test and validate the AI-generated results. Despite these challenges, the advantages of generative AI are huge, and we can expect to see it used more often in the future.
Sources

By conducting scientific research, I write about illness, health and healthcare. As a professional medical writer, my experience includes creating feature articles for newsletters and websites as well as research news stories for doctors and researchers. Reading has been an integral part of me since childhood - I'm fan of "Friends" and the "Harry Potter series". Before this career, I was employed by a French multinational company. However, my passion for reading led me to pursue writing professionally; my first Amazon-published short story entitled "The envelope that changed our lives" has recently been released. In my free moments, I enjoy long bike rides around town.