Generative Ai in Material Science Market is estimated to be USD 8,486 Mn by 2032 with a CAGR of 29.8%
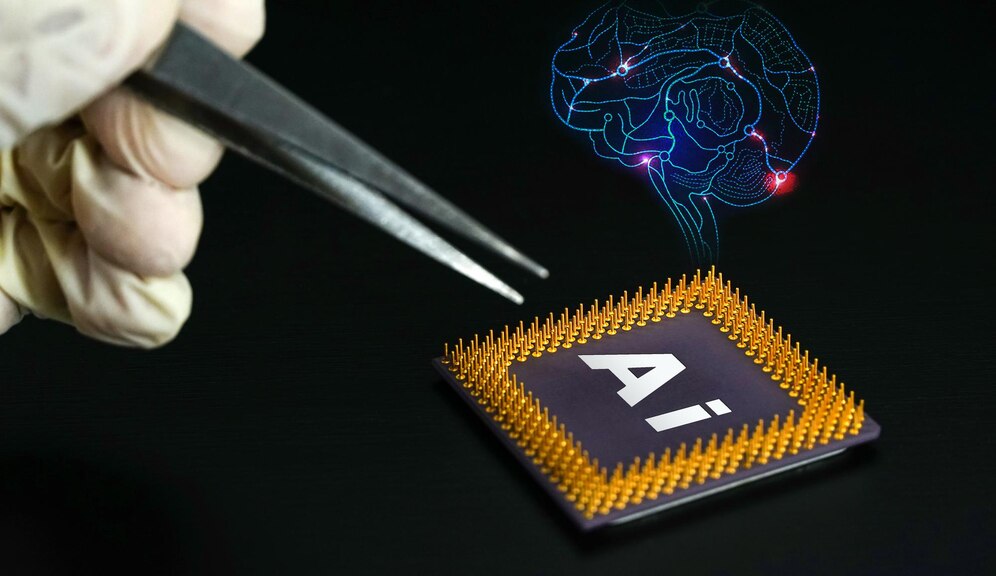
Page Contents
Market Overview
Published Via 11Press : Generative Ai in Material Science Market size is expected to be worth around USD 8,486 Mn by 2032 from USD 667 Mn in 2022, growing at a CAGR of 29.8% during the forecast period from 2022 to 2032.
Material science research has seen remarkable momentum from the application of generative AI over recent years, dramatically altering how researchers design and create advanced materials with enhanced properties. Generative AI refers to using machine learning algorithms to generate novel designs based on predetermined constraints and objectives; its use holds immense promise for expediting material research and development as well as creating breakthrough innovations.
Generative AI offers material science a powerful advantage: exploring vast design spaces and pinpointing optimal materials compositions and structures. Through algorithms like GANs and deep reinforcement learning, researchers can generate and assess many material candidates within a short span of time – significantly speeding up materials discovery which had historically relied upon laborious trial-and-error experimentation.
Generative AI also facilitates the discovery of unconventional and innovative material designs not considered using conventional approaches. By harnessing AI algorithms, researchers can move away from rigid design paradigms and uncover materials with unexpected properties and functionalities – including increased mechanical strength, thermal conductivity, electrical conductivity, optical properties and so forth. This opens up exciting new avenues of research.
Generative AI also facilitates material properties optimization through iteratively refining and fine-tuning designs, enabling researchers to rapidly optimize material properties for specific applications such as energy storage, catalysis, electronics or biomedical devices. Researchers can input specific performance targets or constraints into an AI model which generates material designs meeting them quickly – an iterative process allowing rapid optimization for applications like energy storage, catalysis, electronics, or biomedical devices.
Generative AI in material science is driving collaboration among researchers and industry partners, by using AI-powered design tools companies can streamline research and development efforts, reduce costs and bring innovative materials faster to market. Furthermore, this collaboration between academia and industry helps foster knowledge exchange, technology transfer and commercialization of new materials.
Though generative AI remains widely adopted in material science, significant challenges still stand in its way. Finding high-quality data to train AI models and providing computational resources necessary for complex simulations and calculations remain key obstacles to its widespread adoption. Furthermore, interpretability and explainability must also be ensured to build trust during material development processes.
Request Sample Copy of Generative Ai in Material Science Market Report at: https://marketresearch.biz/report/generative-ai-in-material-science-market/request-sample
Key Takeaways
- Generative AI for material science enables faster discovery and optimization of advanced materials.
- Exploration of unconventional material designs that lead to breakthrough innovations.
- Generative AI allows for fast optimization of material properties for specific applications.
- Collaboration between researchers and industry partners is enhanced through AI-powered design tools.
- High-quality data and computational resources pose obstacles to widespread adoption.
- Trust and reliability must be assured through AI-generated designs which can be understood. Therefore, its interpretability and explainability must be prioritized in their creation.
- Generative AI offers excellent properties and functionalities in advanced materials.
- Continued advances and collaboration should fuel the growth of generative AI in material science markets.
Regional Snapshot
- North America and, specifically, the United States is an epicenter for generative AI innovation in material science research and development. Renowned institutions like MIT, Stanford University, and the University of California Berkeley are at the forefront of research and development efforts related to this subject area. Furthermore, leading tech companies such as IBM, Google and Microsoft are investing heavily in this technology for material innovation applications, helping advance advancement and adoption rates further.
- Europe is making strides forward when it comes to artificial intelligence in material science research, with countries like Germany, the UK and France playing active roles. The European Commission has acknowledged AI's significance by funding collaborative projects using this technique. Furthermore, several universities and research institutes across Europe have established centers or initiatives focused on using AI for materials discovery and design.
- Asia-Pacific region, home to technological powerhouses such as China, Japan and South Korea is witnessing explosive growth in generative AI applications in material science. China in particular has made significant investments in research and development programs dedicated to AI-enabled material innovation such as its National Key Research and Development Program focusing on material sciences research. Strong manufacturing capabilities and emphasis on technological advancement make the Asia-Pacific region a critical player in this arena.
- Latin America is gradually adopting artificial intelligence-powered materials science research, with Brazil and Argentina serving as pioneers. Research institutions and universities throughout these nations are exploring AI algorithms as potential materials optimization solutions in areas like renewable energy production or sustainable materials used.
- Middle East and Africa are witnessing a growing interest in applying generative artificial intelligence (AI) techniques in material science research. Universities and research centers from countries like Israel, Saudi Arabia and South Africa are actively involved in materials research using AI methods; industries like energy, aerospace and healthcare offer ample opportunity for AI-driven materials development in this region.
For any inquiries, Speak to our expert at: https://marketresearch.biz/report/generative-ai-in-material-science-market/#inquiry
Drivers
Accelerated Materials Discovery
Generative AI offers great promise to accelerate materials discovery by rapidly creating and evaluating an immense pool of material candidates. This reduces time-consuming trial-and-error experimentation while opening doors to novel materials with enhanced properties.
Design Optimization
Generative AI allows for the optimization of material designs by iterative refining and fine-tuning their properties. Researchers can input specific performance targets or constraints into an AI model, which then produces designs that meet those requirements. This iterative process enables rapid optimization of materials for specific applications resulting in increased performance and efficiency.
Breakthrough Innovations
Generative AI holds tremendous promise to unleash breakthrough innovations. By exploring unconventional and novel material designs, AI can identify materials with remarkable properties and functionalities not otherwise considered using traditional approaches; this opens up exciting new avenues for developing materials with superior mechanical, thermal, electrical, optical, or any combination thereof properties.
Collaboration and Knowledge Exchange
Generative AI's use in material science fosters collaboration between researchers and industry partners, streamlining research efforts while cutting costs. AI-powered design tools also streamline R&D efforts while streamlining knowledge exchange among academia and industry partners – speeding technology transfer while aiding the commercialization of new materials.
Restraints
Data Access and Quality Analysis Services Provider
Generative AI models rely heavily on accessing high-quality training data. Material science labs often conduct complex and costly experiments that require large numbers of datasets with diverse characteristics to train these AI algorithms effectively and reliably, so inadequate or biased datasets may impede the success and reliability of generative AI algorithms.
Computational Resources
Generative AI algorithms that involve deep learning and complex simulations require considerable computational resources. Training and running AI models for material design and optimization requires access to high-performance computing infrastructure; limited computational resources may pose a barrier to their widespread adoption in material science.
Interpretability and Explainability
Generative AI models often exhibit black-box behavior, meaning their decision-making processes are difficult to interpret or explain. This raises serious concerns over the trustworthiness and reliability of material generated by AI; successfully overcoming this hurdle will facilitate acceptance and adoption in safety-critical applications or industries.
Integration and Experimental Validation
Generic AI may offer promising material designs, but experimental validation is necessary to validate them and assess their performance and feasibility. Integrating AI-driven material design processes with experimental validation processes can be complicated and time-consuming; successfully closing the gap between virtual predictions and real material properties presents unique challenges that must be met for successful implementation.
Opportunities
Advanced Materials for Specific Applications
Generative AI offers unprecedented possibilities for the design and development of advanced materials tailored specifically for specific applications. By employing AI algorithms, researchers can optimize materials with specific properties for industries such as energy storage systems, electronic devices, healthcare implants and lightweight structural materials – leading to more efficient energy storage systems, high-performance devices, personalized implants for biomedicine as well as lightweight structures.
Sustainable Materials Development
Integrating artificial intelligence (AI) in material science can play an invaluable role in creating sustainable materials. AI algorithms can optimize materials to increase energy efficiency, decrease environmental impact and enhance recyclability – aligning with growing consumer demand for eco-friendly materials while helping transition towards a more circular economy.
Materials Informatics
Generative AI in material science can be combined with materials informatics approaches to create powerful tools for materials discovery and design. By using AI algorithms with large databases of materials or computational models, researchers can quickly find new materials with desired properties – making AI and materials informatics an invaluable asset in materials science and engineering research. Together these two fields may result in significant advancements in materials science and engineering research.
Personalized Materials
Generative AI facilitates the design and production of customized materials tailored specifically to individual needs and preferences. By taking into account specific criteria like biocompatibility, durability, or aesthetics, AI algorithms can create material designs that meet these personalized requirements – opening up possibilities for consumer products with tailored features, personalized medicine treatments and adaptive materials for various industries.
Take a look at the PDF sample of this report: https://marketresearch.biz/report/generative-ai-in-material-science-market/request-sample
Challenges
Access and Sharing of Data
Accessing high-quality materials data is essential for training accurate generative AI models, but often dispersed across various research groups, institutions, and industries limiting accessibility and sharing. Achieve data collaboration through standard formats, protocols, platforms or protocols must therefore be addressed as soon as possible.
Ethical and Responsible AI Use
Ethics must be carefully considered when using generative AI for materials research. Biased training data, responsible handling of intellectual property rights and potential misuse of AI-generated designs must all be considered before using this form of artificial intelligence in materials research. Therefore, developing guidelines, regulations and ethical frameworks regarding its usage is of utmost importance.
Scalability and Generalization
Generative AI models must demonstrate scalability and generalization capabilities to be successful in real-world applications. Scaling AI models to accommodate larger datasets while still generalizing across materials systems presents technical challenges – but overcoming them is necessary if one wishes to employ this form of artificial intelligence for material science applications.
Cost and Infrastructure
Implementing generative AI models may require substantial investments in computational resources, infrastructure, and expertise – particularly high-performance computing systems, AI hardware accelerators and highly trained personnel – but can often prove prohibitively expensive for smaller research groups and organizations.
Market Segmentation
By Type
- Materials Discovery and Design
- Predictive Modeling and Simulation
- Process Optimization
Byย Application
- Pharmaceuticals and Chemicals
- Electronics and Semiconductors
- Energy Storage and Conversion
- Automotive and Aerospace
- Construction and Infrastructure
- Consumer Goods
- Other
By Deployment
- Cloud-Based
- On-Premises
- Hybrid
Key Players
- IBM Corporation
- Google DeepMind
- OpenAI
- Kebotix
- Matter
- MIT's Materials Project
- Schrodinger
- Other
Report Scope
Report Attribute | Details |
Market size value in 2022 | USD 667 Mn |
Revenue Forecast by 2032 | USD 8,486 Mn |
Growth Rate | CAGR Of 29.8% |
Regions Covered | North America, Europe, Asia Pacific, Latin America, and Middle East & Africa, and Rest of the World |
Historical Years | 2017-2022 |
Base Year | 2022 |
Estimated Year | 2023 |
Short-Term Projection Year | 2028 |
Long-Term Projected Year | 2032 |
Recent Developments
- In 2021, Google AI researchers developed Morpheus, an innovative new generative AI model capable of designing new materials with desired properties. Morpheus was trained on over 100,000 known materials and can generate novel materials with properties not present anywhere else in nature.
- In 2022, Atomwise unveiled their innovative artificial intelligence platform Atomwise Materials which can assist researchers with creating new materials for various applications. Utilizing both machine learning and quantum mechanics techniques, Atomwise Materials uses predictive modeling of properties for these new materials.
- In 2023, Recursion Pharmaceuticals announced it is using artificial intelligence to discover new medicines for Alzheimer's and Parkinson's diseases. Recursion's AI platform generates drug candidates which are more likely to be effective and have less side effects than traditional methods for drug discovery.
FAQ
1. What Is Generative AI in Material Science?
A. Generative AI in material science refers to the application of machine learning algorithms to design new materials or optimize existing ones, using techniques such as deep learning or generative models for faster material discovery, design optimization, and breakthrough innovation.
2. How does Generative AI accelerate material discovery?
A. Generative AI algorithms enable researchers to rapidly generate and evaluate multiple material candidates with improved properties more quickly, eliminating trial-and-error experimentation while saving both time and effort in their search for materials with enhanced properties and functionalities. By exploring a wide design space, this method enables faster identification of materials with enhanced properties than ever before.
3. What advantages could generative AI offer material sciences?
A. Generative AI has numerous applications in material science, from designing advanced materials for energy storage applications to electronics, healthcare and aerospace. Furthermore, generative AI can contribute to sustainable materials development projects as well as personalized material properties optimization to address individual uses.
4. How can generative AI optimize material designs?
A. Generative AI algorithms are useful tools for iteratively refining material designs based on specific performance targets or constraints, optimizing material properties to meet these objectives and leading to enhanced performance and efficiency. AI models can generate and evaluate various designs until reaching optimal material properties that achieve goals set forth by their creators – ultimately leading to improved performance and efficiency.
5. What are the challenges associated with implementing generative AI in material science?
A. Generative AI presents various challenges, such as access and quality of data for training models, significant computational resource requirements, interpretation/explainability/validity concerns for AI-generated designs generated from material science research as well as integration into experimental validation protocols. Navigating these obstacles successfully is essential to its deployment within material science research.
6. Can AI contribute to sustainable materials development?
A. Generative AI can play an essential role in sustainable materials development by optimizing materials to increase energy efficiency, decrease environmental impact and promote increased recyclability. AI algorithms may even assist with identifying eco-friendly materials to support increased circular economy practices.
7. How does Generative AI facilitate collaboration between researchers and industries?
A. Generative AI-powered design tools streamline research and development efforts, cutting costs while streamlining collaboration between researchers and their industry counterparts. Leveraging AI's capabilities enables researchers and industries to exchange knowledge, speed technology transfer, and facilitate the commercialization of new materials.
Contact us
Contact Person:ย Mr. Lawrence John
Marketresearch.Biz
Tel:ย +1 (347) 796-4335
Send Email:ย [email protected]
Content has been published via 11press. for more details please contact at [email protected]

The team behind market.us, marketresearch.biz, market.biz and more. Our purpose is to keep our customers ahead of the game with regard to the markets. They may fluctuate up or down, but we will help you to stay ahead of the curve in these market fluctuations. Our consistent growth and ability to deliver in-depth analyses and market insight has engaged genuine market players. They have faith in us to offer the data and information they require to make balanced and decisive marketing decisions.